The Ethical Dilemmas of Data Science: Balancing Privacy and Innovation
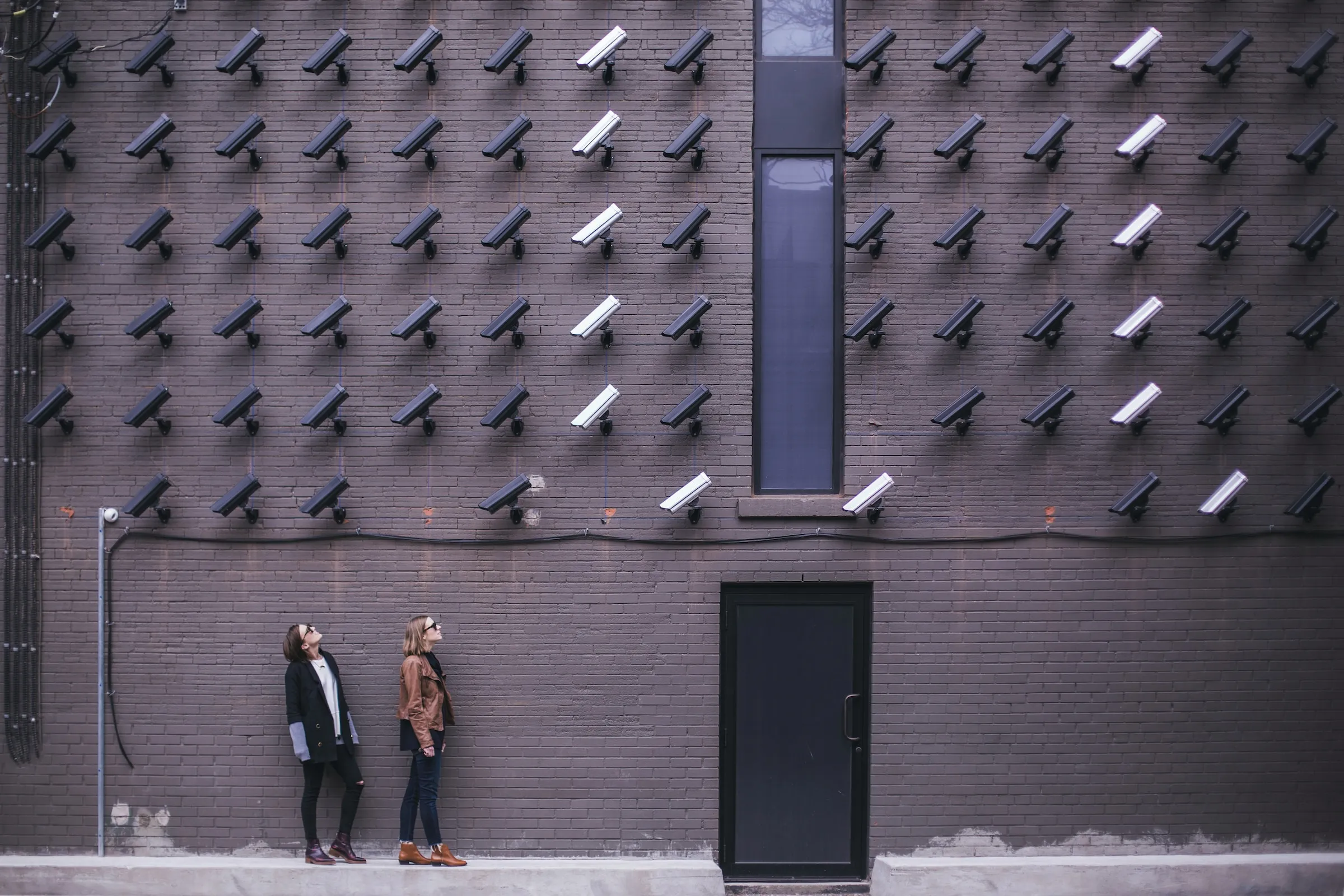
In recent years, the field of data science has propelled industries and businesses into uncharted territories, revolutionising how we make decisions, solve problems, and innovate. However, alongside these immense benefits come pressing ethical concerns that require careful consideration. This article will delve into the ethical dilemmas of data science, examining privacy, data security, bias, fairness, transparency, explainability, accountability, and governance, as well as how to strike a balance between innovation and ethics.
Privacy and data security
Data privacy and security are at the forefront of ethical concerns in data science. The proliferation of data collection has led to a surge in cyberattacks and data breaches, with the annual cost of cybercrime reaching a staggering $10 trillion globally by 2025. As data scientists, we must ensure that the data we handle is protected and used responsibly. For instance, in 2018, the European Union implemented the General Data Protection Regulation (GDPR), which mandated stricter data protection measures and granted individuals more control over their personal information.
Moreover, we must consider the potential harms resulting from the misuse of sensitive data. In 2016, the Facebook-Cambridge Analytica scandal unfolded, revealing that personal data of millions of Facebook users had been harvested without consent to target political advertising. This incident highlighted the need for robust data privacy frameworks and responsible data handling practices.
Bias and fairness
Data science has the potential to inadvertently perpetuate societal biases and inequalities, with biased data feeding into biased algorithms. A striking example of this issue is found in the case of the COMPAS recidivism algorithm. A 2016 investigation by ProPublica revealed that the algorithm incorrectly labelled Black defendants as high-risk at nearly twice the rate of white defendants. This demonstrates how algorithmic bias can exacerbate existing disparities, undermining fairness in decision-making processes.
To address this issue, data scientists must adopt a proactive stance in identifying and mitigating biases in data and algorithms. Techniques such as fairness-aware machine learning, which aims to minimise discriminatory outcomes, are essential for ensuring equitable treatment of different social groups.
Transparency and explainability
The complexity of data-driven models can hinder transparency and explainability, making it difficult for stakeholders to understand how decisions are made. This lack of clarity can lead to mistrust and hinder the adoption of innovative solutions. In 2018, the European Union’s GDPR introduced the “right to explanation”, which grants individuals the right to know how decisions that impact them are made by automated systems.
To increase transparency and explainability, data scientists must work towards creating interpretable models and providing clear documentation of the processes and decisions involved in their creation. Techniques such as local interpretable model-agnostic explanations (LIME) and counterfactual explanations can aid in demystifying complex algorithms and fostering trust.
Accountability and governance
Accountability and governance in data science pertain to determining who is responsible for the consequences of data-driven decisions and ensuring that ethical standards are upheld. In the wake of high-profile data scandals, calls for stronger data governance frameworks have grown louder. In response, organisations such as the Open Data Institute (ODI) and the Partnership on AI have emerged, offering guidelines for ethical data use and promoting collaboration between stakeholders.
Organisations must establish clear guidelines for data use, foster a culture of ethical responsibility, and ensure that consequences for breaches are enforced. Additionally, third-party audits and certifications can help to demonstrate commitment to ethical data practices and build trust among stakeholders.
Balancing innovation and ethics
While the potential of data science to drive innovation is undeniable, striking a balance between innovation and ethical considerations is crucial. This balance involves adopting a responsible and proactive approach to data privacy, security, bias, fairness, transparency, explainability, accountability, and governance. By addressing ethical concerns head-on, data scientists can ensure that the benefits of innovation are not overshadowed by unintended consequences.
Recommendations for ethical data science practices
To promote ethical data science practices, several key steps can be taken by organisations and practitioners:
- Data privacy by design: Implement data protection measures from the outset, ensuring that privacy is an integral part of the data science process.
- Bias detection and mitigation: Be vigilant in identifying and addressing biases in data and algorithms, adopting fairness-aware machine learning techniques where necessary.
- Promote transparency and explainability: Strive to create interpretable models and provide clear documentation of the processes and decisions involved in their development.
- Establish accountability and governance structures: Develop robust data governance frameworks, enforce consequences for ethical breaches, and consider third-party audits and certifications to demonstrate commitment to ethical data practices.
- Foster a culture of ethical responsibility: Encourage a culture of ethical awareness and responsibility among data scientists, providing training and resources on the ethical implications of data science.
- Collaborate with stakeholders: Engage with stakeholders from diverse sectors and backgrounds, ensuring that a broad range of perspectives is considered in the development of ethical data science practices.
Conclusion: The future of ethical data science
The ethical dilemmas of data science pose complex challenges that require a collective effort from industry, academia, and policymakers. By embracing ethical considerations and implementing responsible data science practices, we can harness the immense potential of data-driven innovation while safeguarding the privacy, fairness, and trust of individuals and society.
As the field of data science continues to evolve, it is crucial that ethical guidelines and practices adapt to emerging technologies and societal concerns. By fostering a culture of ethical responsibility and continuously refining our approach to data science, we can navigate the delicate balance between privacy and innovation, ensuring a brighter and more equitable future for all.
Ready to make your data actionable? Talk to us